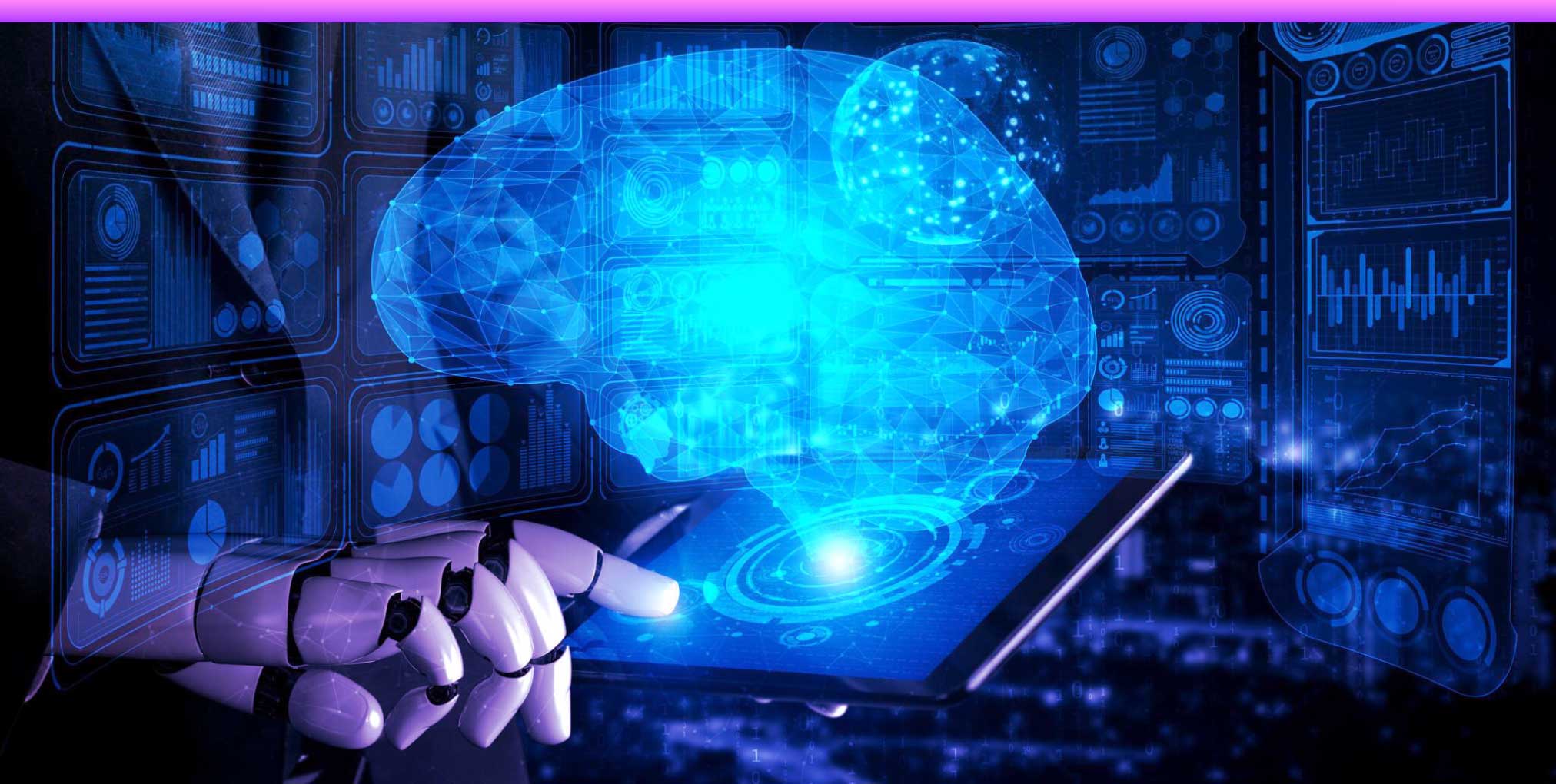
January 26, 2024
When most people think about what machine learning is, it doesn’t seem very clear. It seems like something from the future, with robots taking over. But the truth is that machine learning is already a part of our daily lives, even if we don’t realize it. It’s helping us in many ways, improving things and making some tasks in business and other industries faster and more efficient. It’s also finding patterns that humans might miss.
Machine learning is a $21 billion worldwide industry, and experts predict it will grow to $209 billion by 2029. But what exactly are the use cases of machine learning? Let’s explore 10 uses of machine learning and understand the diverse applications in our daily lives.
One of the prominent examples of machine learning is personalized recommendations. Whether browsing through Netflix, Spotify, or your favorite e-commerce platform, machine learning algorithms analyze your past behavior to suggest movies, music, or products according to your preferences. This use case of machine learning enhances user experience by providing content that aligns with individual tastes.
Personalized recommendations highlight the power of machine learning to transform digital experiences. By understanding individual behaviors and preferences, machine learning algorithms enhance the overall quality of interaction with various platforms. This boost makes content discovery more enjoyable and underlines the user-centric uses of machine learning in our technologically driven world.
Fraud detection in banking is one of the vital use cases of machine learning. Smart algorithms protect our financial transactions from unauthorized and fraudulent activities. This sophisticated use of technology involves deploying machine learning algorithms to explore vast amounts of transaction data for irregularities and suspicious patterns.
In the traditional banking system, manually monitoring every transaction is time-consuming. This is where machine learning steps in, bringing efficiency and accuracy to the forefront. The algorithms are trained on historical data, learning to recognize patterns associated with legitimate transactions and those indicative of potential fraud.
The machine learning algorithms are silently at work when you make a transaction—be it withdrawing cash from an ATM, swiping your credit card, or conducting an online transfer. They analyze a multitude of factors, including:
These algorithms create a baseline of your usual transaction behavior, establishing what you consider’ normal’. For example, if you typically make purchases within your local area and suddenly there’s an attempt to use your card in a different country, machine learning algorithms can identify this as unusual behavior.
Within the vast landscape of healthcare, machine learning emerges as a pivotal player in diagnostics. Specifically, machine learning algorithms have proven instrumental in analyzing medical images, such as X-rays and MRIs, to help doctors identify abnormalities and ensure accurate diagnoses. This application shows the immense potential of machine learning and highlights its life-saving impact in medicine.
When trained on big datasets of medical images, machine learning algorithms excel at recognizing complex patterns and subtle changes that can escape the human eye. These algorithms can quickly process and analyze massive volumes of medical imaging data, providing healthcare professionals valuable insights.
One real-life example is the use of machine learning in breast cancer diagnostics. Algorithms have been developed to analyze mammograms and identify patterns associated with early signs of breast cancer.
In neuroimaging, machine learning has been employed to analyze MRI scans to identify markers of neurological disorders, such as Alzheimer’s disease.
The potential life-saving impact of machine learning in healthcare diagnostics extends beyond the detection of diseases. Machine learning algorithms can expedite the diagnostic process in emergencies, such as identifying signs of a stroke in brain imaging.
As we observe the current ML and AI trends, it’s clear that they’re not just ideas on paper. They’re real tools that make emergency medical responses work better. As ML and AI improve, we can expect a future where technology and healthcare come together even more, helping with quick and accurate diagnoses.
Virtual personal assistants, exemplified by well-known entities like Siri and Alexa, are classic and highly visible examples of machine learning applications. These AI-driven systems show the dynamic nature of machine learning.
ML algorithms continuously learn from user interactions to adapt and improve responses over time. These virtual assistants are not just conveniences; they represent tangible use cases of machine learning in our daily lives.
Machine learning plays a crucial role in the speech recognition capabilities of virtual assistants. These systems employ algorithms that learn to understand and interpret various accents, tones, and languages, making them more versatile and user-friendly.
One of the impressive aspects of virtual personal assistants is their ability to understand context. Machine learning algorithms analyze conversation patterns, enabling assistants to maintain context across multiple queries. For example, if a user asks, “Who is the president?” and follows up with, “Tell me about him,” the assistant can comprehend the continuity and provide relevant information.
Virtual personal assistants heavily rely on Natural Language Processing, a subfield of machine learning, to understand and respond to user inputs. Studying diverse language patterns makes these systems more proficient in generating human-like responses.
Whether setting reminders, answering questions, or controlling smart home devices, virtual personal assistants demonstrate the tangible benefits of machine learning to enhance user experiences in the digital age.
In the manufacturing sector, machine learning is an invaluable asset through its application in predictive maintenance. These uses of machine learning involve analyzing data collected from sensors and equipment, empowering machine learning models to predict when machinery is likely to fail. Implementing predictive maintenance allows for timely interventions and significantly reduces downtime.
Machine learning models analyze data streams from sensors embedded in manufacturing equipment. These models can predict potential faults, such as subtle changes in patterns, temperatures, vibrations, or other indicators. This early detection capability is crucial to predictive maintenance, allowing for proactive measures.
Predictive maintenance results in cost savings by reducing unplanned downtime and preventing costly repairs. By addressing issues in their early stages, manufacturers can extend the lifespan of machinery and avoid the need for expensive emergency repairs or replacements.
Machine learning, specifically through Natural Language Processing, has brought a significant shift in customer service. This application of machine learning is most evident in the deployment of chatbots and virtual assistants. These chatbots have sophisticated algorithms capable of understanding and responding to customer queries in real-time. This example highlights the use cases of machine learning, showing how technology can improve customer support by providing quick and precise assistance.
ML algorithms enable machines to comprehend and interpret human language. In customer service, chatbots equipped with NLP can understand customer queries, regardless of how they are phrased. Whether it’s a specific question about a product or a general inquiry, the system can extract meaning and intent from the language used.
One of the significant advantages of integrating machine learning into customer service is the ability to provide instant responses. NLP algorithms can understand customer queries and generate appropriate and contextually relevant responses. This instant responsiveness offers an efficient customer experience.
NLP-driven customer service systems can offer support in multiple languages. The algorithms can translate and comprehend queries in different languages, widening the scope of customer service accessibility. Moreover, the uses of machine learning in customer service align with the evolving expectations of consumers who seek instant and personalized assistance.
Imagine cars that can drive themselves! That’s what self-driving cars are all about, and they’re using machine learning to do it. ML algorithms help these cars see and understand everything around them.
Autonomous vehicles have various sensors, including LiDAR, radar, cameras, and ultrasonic sensors. These sensors generate massive amounts of data, capturing the vehicle’s surroundings in real-time. Machine learning algorithms process this data, identifying and categorizing objects, pedestrians, other cars, and obstacles.
Machine learning enables autonomous vehicles to understand and interpret their environment. This includes recognizing traffic signs, understanding road markings, and identifying complex scenarios like intersections and roundabouts. The algorithms learn from diverse driving scenarios, allowing the vehicle to adapt to various road conditions and situations.
One of the critical aspects of machine learning in autonomous vehicles is its ability to make informed decisions. Algorithms process the data from sensors and assess potential courses of action. For example, they can decide when to change lanes, how to navigate traffic, or when to stop at a traffic signal. This decision-making capability is essential for the safe and efficient operation of self-driving cars.
In the social media world, machine learning plays a crucial role in content moderation, ensuring that online platforms remain safe and welcoming for users. This application involves the use of algorithms to analyze text, images, and videos, pinpointing potentially harmful or inappropriate content. By doing so, machine learning contributes significantly to maintaining a secure online environment and enforcing community guidelines.
Machine learning algorithms scrutinize text content on social media platforms. They are trained to recognize patterns that might indicate hate speech, bullying, or any form of harmful communication. This analysis helps identify and address such content promptly.
Visual content, including images, undergoes a thorough examination by machine learning algorithms. These algorithms can detect inappropriate or offensive images, such as explicit content or graphic violence, adhering to community standards set by the platform.
Videos are subjected to machine learning analysis to identify potential violations of community guidelines. Whether it’s violent scenes, hate speech, or any other prohibited content, algorithms work to flag and remove such videos to maintain a safe online space.
The sheer volume of content shared on social media platforms is immense. Machine learning algorithms can process this content at scale and with speed. This fast process enables platforms to moderate and respond to potential violations in real-time efficiently.
The use cases of machine learning in social media content moderation are essential to creating a safe and inclusive online environment. As technology improves, social media platforms are working to improve how they control and manage content.
In daily email communications, machine learning takes center stage in the important task of filtering out spam. This application involves machine learning algorithms that, through learning from patterns and user feedback, distinguish between legitimate emails and spam. The result is an organized and secure inbox experience, ensuring users can focus on meaningful communications without harm.
Users play a crucial role in refining the effectiveness of email filtering algorithms. When users mark an email as spam or move it to a specific folder, this feedback is incorporated into the algorithm’s learning process. Over time, the algorithm adapts to user preferences and refines its ability to categorize emails accurately.
Email spammers are constantly evolving their tactics. Machine learning algorithms are designed to adapt to these changes. They continually update their understanding of spam patterns, ensuring they can effectively filter out new and emerging forms of spam.
Spam emails often carry security risks, such as phishing attempts or malware. Machine learning in email filtering adds a layer of security by preventing users from interacting with malicious content. By using different types of machine learning, algorithms adapt to evolving patterns, learn from user feedback, and provide users with an organized inbox.
In the fast-moving world of finance, one of the major use cases of machine learning is creating smart trading plans. This means using special computer programs to look at how the market has acted before and what’s happening now. These programs help make quick and smart decisions, make investments better, and predict where the market might go. This way is faster and more accurate than people have traditionally done it.
Machine learning algorithms excel at analyzing vast amounts of financial data, including market trends, stock prices, trading volumes, and economic indicators. These algorithms can identify complex patterns and relationships within this data, providing valuable insights into market dynamics.
Algorithmic trading involves the use of algorithms to execute trading strategies automatically. Machine learning algorithms power these strategies, allowing for rapid execution of trades in response to specific market conditions.
Machine learning contributes significantly to risk management in financial trading. Algorithms assess risk factors, such as market volatility, economic indicators, and historical performance, to optimize investment portfolios and minimize potential losses.
The uses of machine learning in financial trading are bringing a big change. It gives traders and investors robust tools to understand and deal with complicated markets.
For the best Machine Learning Services, turn to Xeven Solutions. We specialize in combining ML and AI to deliver highly efficient solutions. Whether you want the latest technology integration or customized applications, our team at Xeven Solutions is dedicated to providing the best-in-class services to meet your needs. Make your projects stand out with our expertise in Machine Learning Services, bringing innovation and efficiency to the forefront of your solutions.
In conclusion, the 10 uses of machine learning are transforming how we live and work. From personalized recommendations to healthcare diagnostics, machine learning is a driving force behind technological innovation.
As we look ahead, it’s essential to learn about different types of machine learning and keep up with trends in ML and AI. The arrival of Machine Learning Services shows that this technology is used more in other areas. It suggests a future where machine learning keeps making our everyday experiences better.