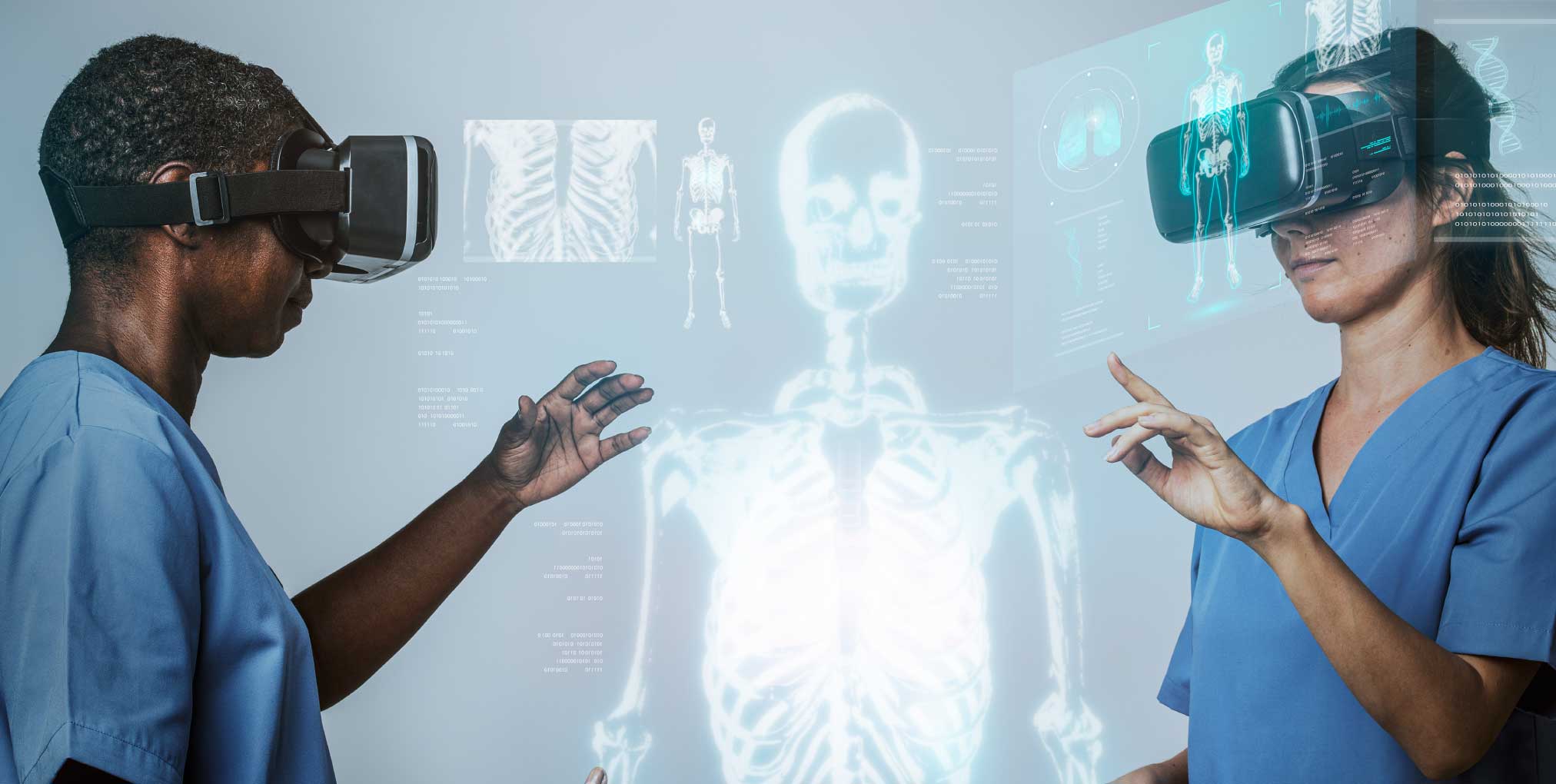
October 2, 2023
In today’s fast-paced world, technology continues to advance astonishingly. Its impact on various industries is undeniable. One of the fields where machine learning services have shown remarkable promise is healthcare. In the healthcare industry, machine learning analyzes large amounts of medical data. As a result, it helps improve more accurate results.
This article will explore how machine learning benefits healthcare. We will explore 10 real-world use cases of ML in healthcare. Also, explore why machine learning in healthcare is essential, its benefits, and recent developments.
Machine learning is a branch of artificial intelligence that focuses on developing algorithms and statistical models. It allows computers to learn from and make predictions or decisions. Decisions are based on data without explicit programming. Machine learning enables computers to improve their performance on a specific task. Accuracy improves with time as they are exposed to more data.
The importance of machine learning in healthcare cannot be overstated. It has the potential to enhance the quality of patient care. As a result, they streamline operations, reduce costs, and even save lives. Here are some key reasons why machine learning is crucial in healthcare: –
Machine learning algorithms can analyze vast datasets. It includes medical records, images, and lab results. It can assist healthcare providers in making accurate and timely diagnoses. It leads to early detection of diseases and more effective treatment plans.
Each patient is unique, and their response to treatments can vary. Machine learning helps tailor treatment plans based on individual patient data. As a result, they are optimizing outcomes while minimizing side effects.
ML can analyze historical patient data. ML models can predict disease outbreaks, patient admissions, and individual patient risks. It enables healthcare organizations to allocate resources more efficiently.
Machine learning accelerates the drug discovery process. It can do it by identifying potential drug candidates and predicting their efficacy. It can significantly reduce the time and cost involved in developing new medications.
Machine learning is instrumental in detecting fraudulent activities in healthcare. Activities like insurance fraud or improper billing. It saves money and ensures that resources are allocated to genuine patient needs.
The AI benefits in healthcare are substantial and far-reaching. Some advantages are given below: –
Machine learning algorithms can analyze large volumes of medical data with high accuracy. As a result, it reduces the risk of misdiagnosis and human errors.
Automation of data analysis leads to faster diagnoses. It is critical in cases where time is of the essence—time-sensitive things like identifying life-threatening conditions.
Efficiency gains through ML can result in significant cost savings for healthcare organizations. As a result, it benefits patients by reducing the cost of care.
ML models can identify patterns and risk factors that humans might overlook. As a result, it enables the early detection of diseases like cancer and diabetes.
Tailoring treatments to individual patients’ needs improve treatment efficacy and minimizes adverse reactions. As a result, it promotes better patient outcomes.
The application of machine learning for healthcare is continually evolving. Recently, Developments of Machine Learning in Healthcare have been groundbreaking: –
NLP techniques have been employed to extract valuable information from unstructured medical records. As a result, it makes it easier for healthcare professionals to access critical patient data.
Machine learning models can predict patient outcomes. It allows healthcare providers to intervene and improve patient care proactively.
Machine learning has shown remarkable success in analyzing medical images. As a result, it aids in the early detection of diseases like cancer. It even predicts patient outcomes based on imaging data.
Machine learning is being used to identify potential drug candidates. It can predict their interactions and streamline the drug discovery process.
Machine learning facilitates remote patient monitoring. It makes telehealth more effective and accessible, especially in times of crisis.
AI and ML have significantly contributed to healthcare. It improves patient engagement, access to information, and the efficiency of administrative tasks. Here are ten real-world use cases of AI and ML in healthcare: –
Machine Learning models can analyze vast datasets, including patient records, medical imaging, and genetic information. By scrutinizing these data, ML algorithms can predict disease risks in individuals. For example, ML can forecast the likelihood of diabetes, heart disease, or cancer, enabling early interventions and lifestyle modifications.
The use of ML in medical imaging has revolutionized the field. It allows for the accurate and swift analysis of X-rays, MRIs, and CT scans. ML algorithms can detect anomalies, such as tumors or fractures. It may be challenging to identify through conventional methods. It accelerates diagnosis and treatment planning, potentially saving lives.
Drug discovery is a time-consuming and expensive process. Machine Learning expedites this by analyzing biological data. It predicts how compounds will interact with diseases. It identifies potential drug candidates. As a result, it leads to more efficient drug development and reduced costs.
One of the significant advantages of AI and ML in healthcare is personalization. ML can analyze a patient’s medical history, genetics, and lifestyle factors. As a result, tailor custom treatment plans. This results in more effective treatments, fewer side effects, and better patient outcomes.
Machine Learning enables remote monitoring of patients, especially those with chronic conditions. Wearable devices and sensors collect data, which is then analyzed by ML algorithms. Healthcare providers can receive real-time updates on their patients’ health. Patients can intervene when necessary and reduce hospital readmissions.
Diabetic retinopathy is a complication of diabetes that damages the eye’s retina. It can lead to blindness if undetected. AI algorithm analyzes retinal scan images. It can detect early signs like microaneurysms and bleeding that humans may miss. It allows more people to be screened and receive timely treatment before vision is affected.
Sepsis is a life-threatening response to infection that can lead to tissue damage, organ failure, or death. By monitoring patterns in a patient’s vital signs, labs, medications, and history tracked in electronic records, AI systems can predict the onset of sepsis up to a day in advance. It gives doctors a head start to intervene with antibiotics and other supportive care to treat sepsis before it worsens.
When cancer spreads from its original site to other parts of the body, it worsens the prognosis and changes treatment. Deep learning algorithms can evaluate digitized slides of lymph node tissue samples taken during cancer operations. The AI detects cancer cells and classifies the lymph nodes, assisting pathologists in cancer staging and planning the most effective therapies.
Not all depression treatments work equally for each patient. AI and machine learning analyze various clinical data to understand factors unique to an individual that may impact how well they respond to different antidepressant medications or therapy approaches. The system then recommends the most personalized treatment path for a patient.
AI helps optimize medical imaging protocols, adjusting settings like radiation dosage, contrast agent levels, and image resolution tailored to a specific patient’s attributes, like age and size. It minimizes health risks from overexposure to radiation while maintaining the image quality needed for accurate diagnosis.
Spending time in the ICU puts stress on the body. AI systems identify patterns in a patient’s history that may indicate a higher risk of readmission within 30 days of discharge. With earlier warnings, doctors can better coordinate follow-up care and support to prevent readmissions.
In emergencies, when patients cannot identify their medications, AI and machine vision can analyze the physical attributes of pills, such as shape, color, and markings, to rapidly determine the drug and dosage. This helps emergency personnel provide the proper treatment.
Wearable devices and remote monitoring tools track patient vital signs and behaviors over time. AI detects any changes in the data early on that may signal worsening symptoms for chronic conditions like heart failure before a crisis occurs. Faster treatment helps avoid costly hospitalizations.
By analyzing prescription drug monitoring program data, AI identifies at-risk patients and prescribing patterns outside guidelines to help address the opioid epidemic. It also flags doctors that may be overprescribing, helping curb misuse, abuse, and overdoses.
Chatbots using natural language processing respond to standard medical questions from patients and the public. They provide health information to address issues and triage non-emergency concerns to reduce strain on clinicians while helping address gaps in rural healthcare access.
This article shows how Machine learning in healthcare has transformed the patient care industry. It can improve accuracy, efficiency, and patient outcomes. AI and ML have led to innovations in diagnostics, treatment, and drug discovery. As technology advances, AI & ML with healthcare synergy promises a brighter and healthier future for all.
Machine learning is vital because it empowers computers to learn from data and make data-driven predictions and decisions. In healthcare, it enhances accuracy, optimizes treatments, and reduces costs, ultimately improving patient care.
Recent developments include predictive analytics for patient outcomes, natural language processing for medical records, and AI-driven drug discovery. These advances improve healthcare efficiency, patient care, and cost-effectiveness, showcasing the increasing benefits of machine learning in the medical field.
Machine learning is used in healthcare for disease diagnosis, personalized treatment plans, predicting patient outcomes, and optimizing medical imaging. It enables the analysis of vast medical data to improve accuracy and efficiency in patient care.
The future of machine learning in healthcare is promising. It will likely involve more advanced applications, including real-time patient monitoring, genomics, drug discovery, and enhanced telemedicine. As AI and ML technologies evolve, they will play an increasingly vital role in revolutionizing healthcare delivery and patient outcomes.