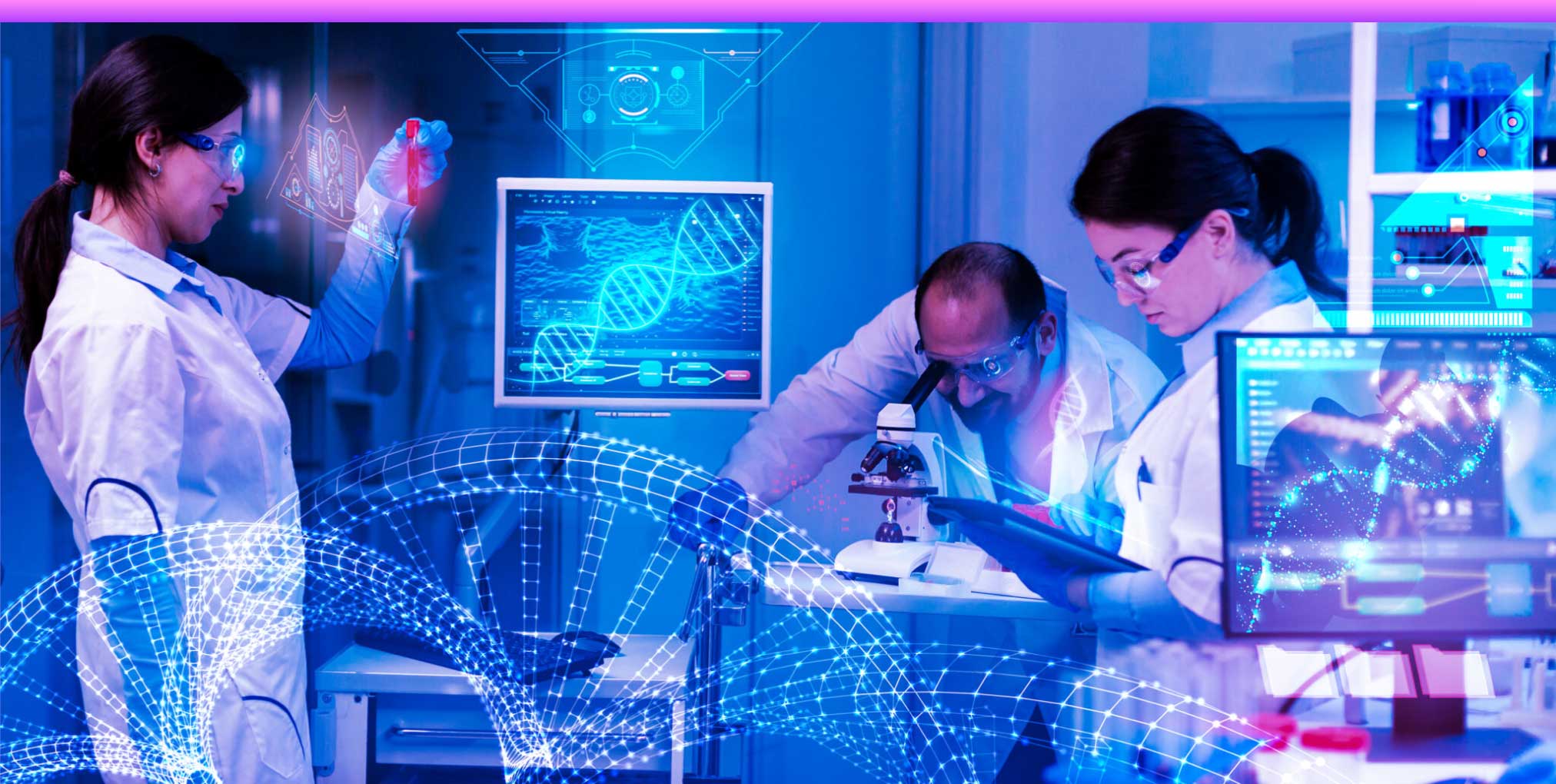
June 13, 2024
Artificial Intelligence (AI) has significantly transformed various industries and continues to impact today’s digital world. The healthcare industry, in particular, has seen rapid adoption of AI technologies to improve patient care and increase efficiency. In recent years, there has been a growing interest in utilizing AI for clinical development, with many pharmaceutical companies integrating this technology into their research and development processes.
With the continued advancements in technology, AI has become a valuable tool in various aspects of clinical development, from drug discovery to patient recruitment and monitoring. This powerful technology can analyze vast amounts of data at an incredible speed, providing valuable insights and predictions that can significantly impact the development of new treatments.
In this blog, we will discuss the application of AI in healthcare, specifically clinical development. We will explore how AI improves processes, enhances decision-making, and drives innovation in the pharmaceutical industry. So, let’s start and discover AI’s potential for clinical development.
AI for clinical development represents a convergence of advanced technology and medical research to accelerate the development of new treatments and therapies. Clinical development involves a series of phases designed to test the safety, efficacy, and optimal use of new drugs or medical devices.
Traditionally, this process is lengthy, complex, and resource-intensive, often taking years and significant financial investment. AI is set to change this landscape by enhancing various aspects of clinical trials, from patient recruitment to data analysis.
AI’s connection to clinical development is complicated. At its core, AI uses vast datasets and complex algorithms to extract meaningful insights that can guide decision-making throughout the clinical trial process.
Machine learning models, for instance, can predict outcomes based on historical data, while natural language processing can sift through scientific literature to identify potential drug candidates. By integrating these AI-driven capabilities, clinical researchers can enhance the precision and speed of their work, ultimately bringing new treatments to market more efficiently.
The integration of AI into clinical development can be traced back to the increasing availability of big data and advancements in computational power. AI’s ability to process and analyze vast amounts of data quickly and accurately has made it a valuable tool in medical research.
Early applications included using machine learning algorithms to predict patient outcomes and identify potential drug candidates. Today, AI’s role has expanded to include;
According to McKinsey & Company research, the adoption of AI for clinical development has emphasized operational excellence and acceleration. However, advances in scientific AI have made this the time to use modern analytical tools and novel data sources to design more precisely.
Drug discovery is a time-consuming and expensive process that can take years and cost billions of dollars. However, AI in clinical development is quickly changing the game by analyzing vast datasets. Machine learning algorithms can sift through millions of chemical compounds to identify potential drug candidates in a fraction of the time it would take a human researcher.
Clinical trials are essential for determining the safety and efficacy of new drugs, but delays, high costs, and low success rates often plague them. AI in clinical trials can address these challenges by optimizing various aspects of the trial process.
AI can simulate different trial designs to identify the most effective one, reducing the likelihood of trial failures. This includes optimizing dosage levels, treatment durations, and patient stratification.
AI-powered tools can monitor patient adherence to the trial protocol in real time, ensuring the data collected is accurate and reliable.
Patient recruitment is one of the most critical and challenging aspects of clinical trials. Traditional methods often rely on manual processes and broad inclusion criteria, leading to delays and under-representation of specific patient populations. AI for clinical development solves these challenges by automating and optimizing the recruitment process.
Machine learning algorithms can analyze electronic health records (EHRs) to identify patients who meet specific inclusion criteria, ensuring a more targeted and efficient recruitment process.
AI can also predict patient eligibility based on historical data, improving the speed and accuracy of patient selection. This accelerates the recruitment phase and enhances the diversity and representativeness of clinical trial participants.
Moreover, AI can help identify patient populations that are traditionally underrepresented in clinical trials, such as minorities and those with rare diseases. By ensuring a more inclusive and diverse patient pool, AI contributes to the development of effective treatments across different demographic groups.
AI clinical trials benefit significantly from predictive analytics, which uses historical data to forecast future outcomes. In clinical development, predictive analytics can identify potential risks and inefficiencies early in the trial process. For example, machine learning models can predict patient dropout rates, adverse reactions, and other critical factors that may impact a trial’s success.
By identifying these risks early, researchers can implement proactive measures to mitigate them, such as adjusting trial protocols or enhancing patient monitoring. This leads to more robust and reliable AI clinical trials, ultimately accelerating the development of new treatments.
Predictive analytics also play a significant role in optimizing trial design. By analyzing past trial data, AI can help researchers determine the most effective trial parameters, such as dosage levels and treatment schedules. This increases the likelihood of trial success and reduces the need for multiple trial iterations, saving both time and resources.
Generative AI is an emerging technology that holds great promise for clinical development. Unlike traditional AI models that rely on existing data, generative AI creates new data sets that can be used to simulate clinical trials. This capability allows researchers to explore various scenarios and hypotheses without requiring extensive and costly real-world trials.
For instance, generative AI can model patient responses to treatment regimens, predict potential side effects, and identify new drug candidates. By providing a virtual testing ground, generative AI in clinical trials can significantly reduce the time and cost associated with traditional trial-and-error methods.
Generative AI also facilitates the development of synthetic control arms in clinical trials. These synthetic control arms can substitute for placebo groups, reducing the ethical concerns associated with withholding treatment from patients.
The volume of data generated during clinical trials is immense, encompassing patient information, treatment outcomes, and biological markers, among other variables. Analyzing this data manually is not only time-consuming but also prone to errors. AI-driven data analysis offers a solution by automating the process and providing real-time insights.
Machine learning algorithms can identify patterns and correlations in complex data sets that human analysts may overlook. This capability enhances the accuracy of data interpretation, leading to more reliable and actionable conclusions.
AI can integrate data from multiple sources, such as genomic data, imaging studies, and clinical records, providing a comprehensive view of patient responses and treatment efficacy.
AI-driven data analysis also facilitates adaptive trial designs, where parameters are adjusted in real-time based on interim results. This allows researchers to refine their hypotheses and continuously improve trial efficiency.
As AI advances, we expect its clinical development applications to grow. Future trends include using AI for personalized medicine, where we tailor treatment plans to individual patient profiles based on genetic, environmental, and lifestyle factors.
AI’s ability to analyze vast amounts of data and identify subtle patterns will be crucial in developing these personalized therapies.
It is likely to play a more prominent role in post-market surveillance, monitoring treatments’ long-term safety and efficacy after they have been approved. AI can identify potential safety issues and inform regulatory decisions by analyzing real-world data from EHRs, social media, and other sources.
However, several challenges remain. Data privacy and security are significant concerns, as clinical trials involve sensitive patient information. Ensuring the ethical use of AI and maintaining patient trust will be critical. Robust data governance frameworks and transparent AI algorithms are essential to address these concerns.
Xeven Solutions’ AI development services can assist in implementing AI in clinical development, providing custom solutions for your specific needs. XevenSolutions will continue to adapt and deliver cutting-edge technology for improved efficiency and outcomes as the field evolves.
Integrating AI for clinical development is changing how new treatments and therapies are developed. From enhancing patient recruitment and predictive analytics to leveraging generative AI and AI-driven data analysis. The benefits of AI in clinical trials are varied.
As technology continues to evolve, AI’s potential to transform clinical development will only grow, offering new hope for faster, more efficient, and more effective medical research. However, addressing the challenges associated with AI implementation will be crucial to fully realizing its potential in this field.
AI’s transformative impact on clinical development is undeniable. It offers a future where medical research is faster, more precise, and more inclusive. We can look forward to a new era of medical innovation that brings life-saving treatments to patients more effectively than ever before.