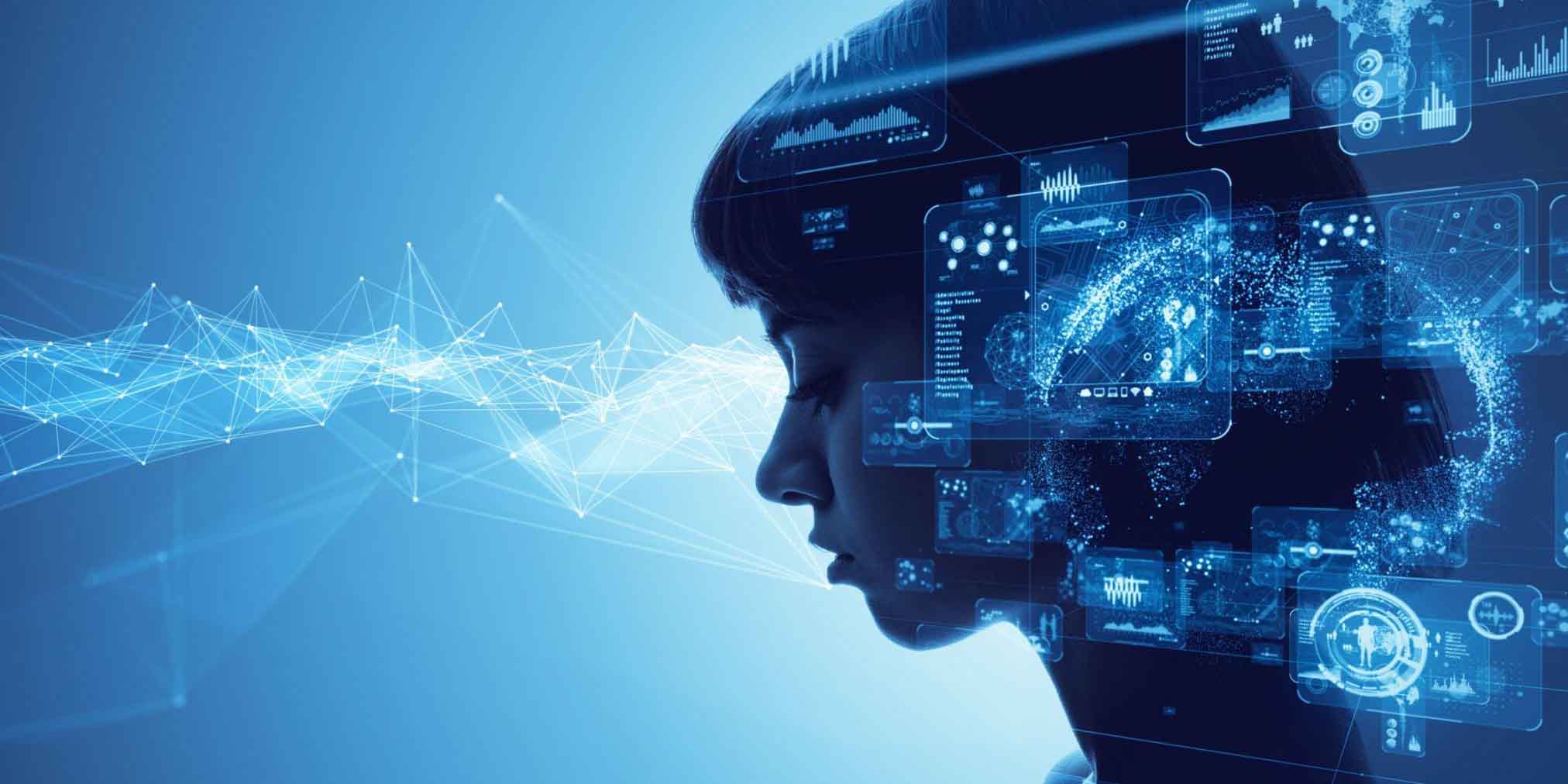
September 5, 2023
Deep Learning and Machine Learning are two of the most popular concepts and tools in Artificial Intelligence (AI). They boast impressive applications and solutions for various industries. Although some may think the two are interchangeable, the two fields differ in several ways. This blog post will explore the key differences between these two technologies and discuss their respective strengths and weaknesses.
Deep Learning is a branch of artificial intelligence that seeks to emulate the ability of the human brain to learn, recognize patterns, and make decisions. It takes data from large and complex datasets and builds models to identify correlations between inputs and outputs.
This allows us to create systems that can more accurately predict results and automate:
Utilizing deep neural networks, Is revolutionizing how computers think and communicate with the world around them by creating smarter decision-making algorithms.
Machine learning is an area of artificial intelligence that enables computers to identify patterns and learn from data without explicit programming. It uses algorithms to analyze large amounts of data, using the information to recognize trends. It can be used for predictive analysis and decision-making.
For example, machine learning development services can help a computer recognize objects in a picture or decide whether a loan should be approved. The potential applications are almost limitless – from detecting cancer in medical images to predicting stock market performance.
With the advent of technologies such as big data, the Internet of Things, and cloud computing, machine learning is poised to become one of the most powerful tools of our time.
Deep Learning involves complex algorithms with multiple layers that collect data through a neural network, allowing the AI to sift through an immense amount of data and find patterns to produce results.
On the other hand, machine learning typically uses simpler algorithms and labeled data to create predictive models that identify different characteristics within existing datasets–this allows less data to be legible while still producing accurate results compared to Deep Learning.
Understanding the differences between these two robust AI solutions could be vital to hitting various business goals in the future.
Deep learning and machine learning generally manipulate data sets to compare outcomes in specific scenarios and develop models based on their insights. However, one key difference between these two fields is their neural network architectures.
Machine learning typically relies on external networks using a few layers, while deep Learning utilizes much larger networks, often composed of many layers. The final decision made by external neural networks with few layers tends to be more strongly affected by each layer’s output due to their limited capacity.
Deep learning models excel at learning intricate patterns due to their numerous hidden layers, enabling seamless information integration between consecutive layers.
One key difference of Deep Learning vs. Machine Learning is their training data requirements. Machine Learning requires relatively small datasets to identify patterns, while Deep Learning requires much larger datasets with labeled input and output data.
Additionally, the training sets used when creating a Deep Learning system must encompass all the variations they will likely encounter while in use. As such, essential to successful Deep Learning is generating massive amounts of high-quality datasets, which can be quite a challenge for many organizations.
Deep Learning and Machine Learning are two primary approaches to AI technology, each serving an essential purpose. One of the key differences between these two approaches is automated feature extraction.
Machine Learning requires manual feature engineering. Here the engineer needs to identify features from the data and map them to an algorithm.
On the other hand, Deep Learning’s automated feature extraction uses its deep neural networks to derive new features from existing ones. This helps discover even more underlying complex relationships within data. It can bring powerful insights that might have been previously unidentified by traditional feature engineering techniques used in Machine Learning.
Deep Learning has proven successful in many industries like healthcare, manufacturing, and retail. It is commonly used for natural language processing and image recognition because it can process large amounts of data sets. For example, it can be used to automate the detection of objects in large-scale medical scans or retail images.
On the other hand, machine learning is best suited for small-scale datasets that don’t require much processing power. It can be used for predictive tasks such as analyzing customer purchase behaviors or making recommendations based on viewing habits. As the explosion of data continues, deep Learning will expand its reach across various industries.
Deep Learning’s powerful AI system can be applied to tasks like pattern recognition, making it ideal for jobs like facial recognition and natural language processing. In terms of consumer-facing products, this could mean applications such as speech recognition, recommendation engines, automated assistants, and more.
On the other hand, machine learning is much better suited to predictive analytics, which means it is heavily used in fraud detection systems, financial forecasting software, and gaming. Consumers will also benefit from its use in dynamic pricing algorithms used by many online vendors today.
Integrated into everyday life apps like voice recognition assistants or automated financial forecasting tools, deep Learning and machine learning have far-reaching implications for consumer-facing technology today.
Deep Learning and machine learning are two powerful tools that have enabled businesses to create innovative products and services. Both technologies continue to advance rapidly and open up a world of possibilities regarding modern customer-facing technology. With advancements in both artificial intelligence technologies, there is no doubt that consumers will benefit from their use for years to come.